Machine Learning Operations (MLOps)
Empower Your Machine Learning with Opsio’s MLOps Solutions and consulting services
Integrate cutting-edge MLOps solutions and MLOps consulting from Opsio to accelerate your machine learning projects and maximize operational efficiency.

Optimize Your AI Capabilities with Azure MLOps
By utilizing our comprehensive MLOps consulting services, you can anticipate quicker model deployment, streamlined workflows, and significantly improved time to market. Opsio empowers your teams to focus on innovation while we handle the complexities of machine learning operations. This allows your data scientists and developers to concentrate on crafting innovative algorithms and extracting valuable insights, rather than managing infrastructure. Our support extends to performance tuning, cost optimization, and ensuring compliance with data privacy regulations, making your ML projects not only faster but also more secure and compliant with global standards.
Deep Dive into MLOps: Unleashing Machine Learning Capabilities
Explore the full potential of machine learning with Opsio’s machine learning operations expertise. Our approach to MLOps as a service ensures that your projects are not only deployed but also maintained and scaled with precision. This comprehensive service is designed to bridge the gap between the rapid development of ML models and the operational capabilities required to support them in production. By standardizing and automating the ML lifecycle, we facilitate faster deployments, more reliable performance, and greater scalability. This process helps mitigate the common challenges associated with deploying machine learning models, such as dependency management and environment discrepancies between development and production.

Furthermore, our commitment to managed MLOps means we are always ahead of the curve, implementing the latest ML techniques and tools to ensure your operations are efficient and your models are accurate. This proactive approach includes continuous integration and delivery (CI/CD) for machine learning models, enabling iterative and responsive development that can quickly adapt to new data and evolving business requirements. Partner with Opsio to transform your machine learning landscape and achieve outstanding results in your industry. Our managed MLOps service provides not just technical expertise but also strategic guidance to ensure that your machine learning initiatives align with your overall business objectives, driving innovation and competitive advantage.
Advanced AWS MLOps Integration for Enhanced Machine Learning Efficiency
With Opsio, you gain access to advanced tools and methodologies that refine your ML lifecycle from development to production. Our machine learning for IT operations strategy optimizes every phase of your ML projects, ensuring they deliver actionable insights and tangible business value. We focus on automating the ML pipeline, implementing robust version control, and providing detailed analytics that help you measure and improve the impact of your ML models. Additionally, our proactive management of the AWS infrastructure ensures that your machine learning operations are cost-effective, highly available, and continuously optimized for changing business needs.
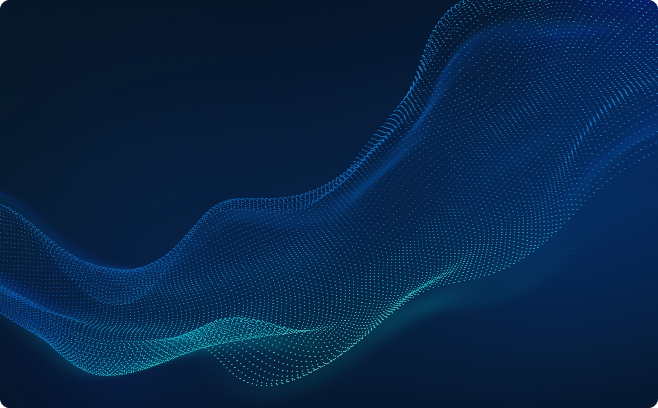
Certified AWS expertise,
Available 24/7
Transforming Machine Learning Operations with Advanced MLOps
Our focus on creating a seamless ecosystem where your data scientists and IT teams can collaborate effectively is crucial for driving innovation and value. This collaborative environment enables quick ideation, experiment, and deployment cycles, which are essential for the dynamic field of machine learning. By facilitating a close integration of various teams, we help ensure that the machine learning models developed are not only innovative but also practically deployable and scalable within your existing IT infrastructure. This harmonious integration reduces the friction typically encountered when moving projects from research to production, thereby accelerating the time-to-value of your machine learning investments.
Moreover, our MLOps services go beyond deployment to include rigorous monitoring and management of your machine learning models in production. This includes implementing model versioning, performance tracking, and automatic retraining policies to deal with model drift over time. Our advanced monitoring frameworks also help identify and rectify issues before they affect your operational efficiency, ensuring that your machine learning systems perform optimally under various conditions. With Opsio, you gain a reliable partner who not only understands the technical landscape of machine learning but also appreciates the business impact of these advanced analytical tools, helping you leverage AI and machine learning to its fullest potential.
Stay Ahead of the Cloud Curve
Get monthly insights on cloud transformation, DevOps strategies, and real-world case studies from the Opsio team.
WHY CHOOSE OPSIO FOR MLOPS
Choose One Approach Or Mix And Match For Maximum Efficiency And Results.
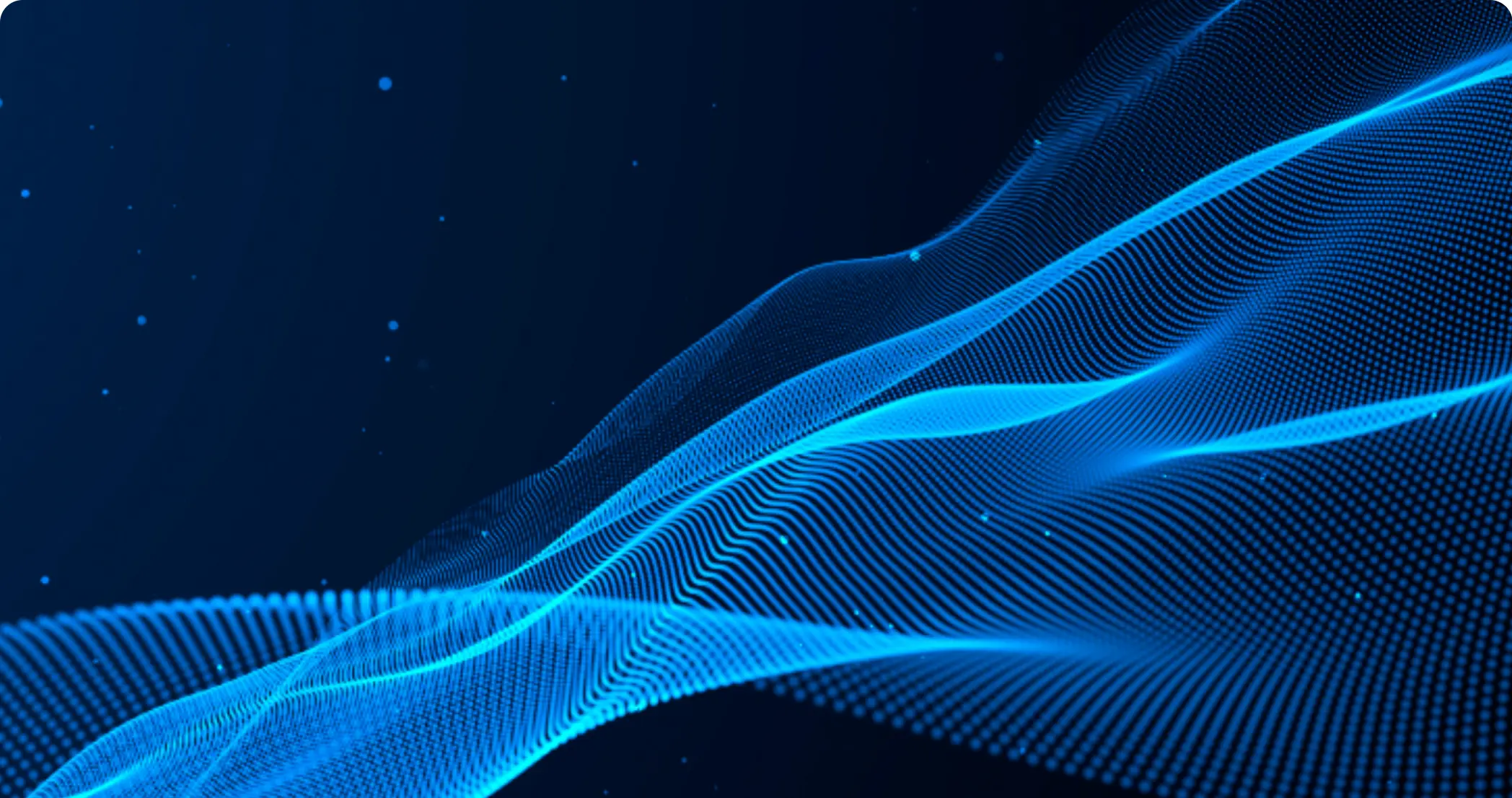
Faster Deployment
Accelerate the time from model development to deployment.
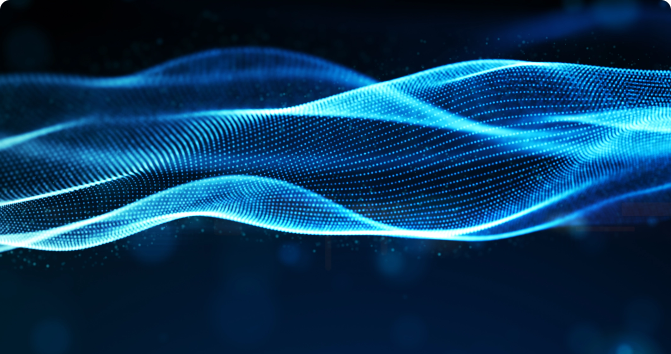
Increased Efficiency
Reduce operational costs and resource usage.
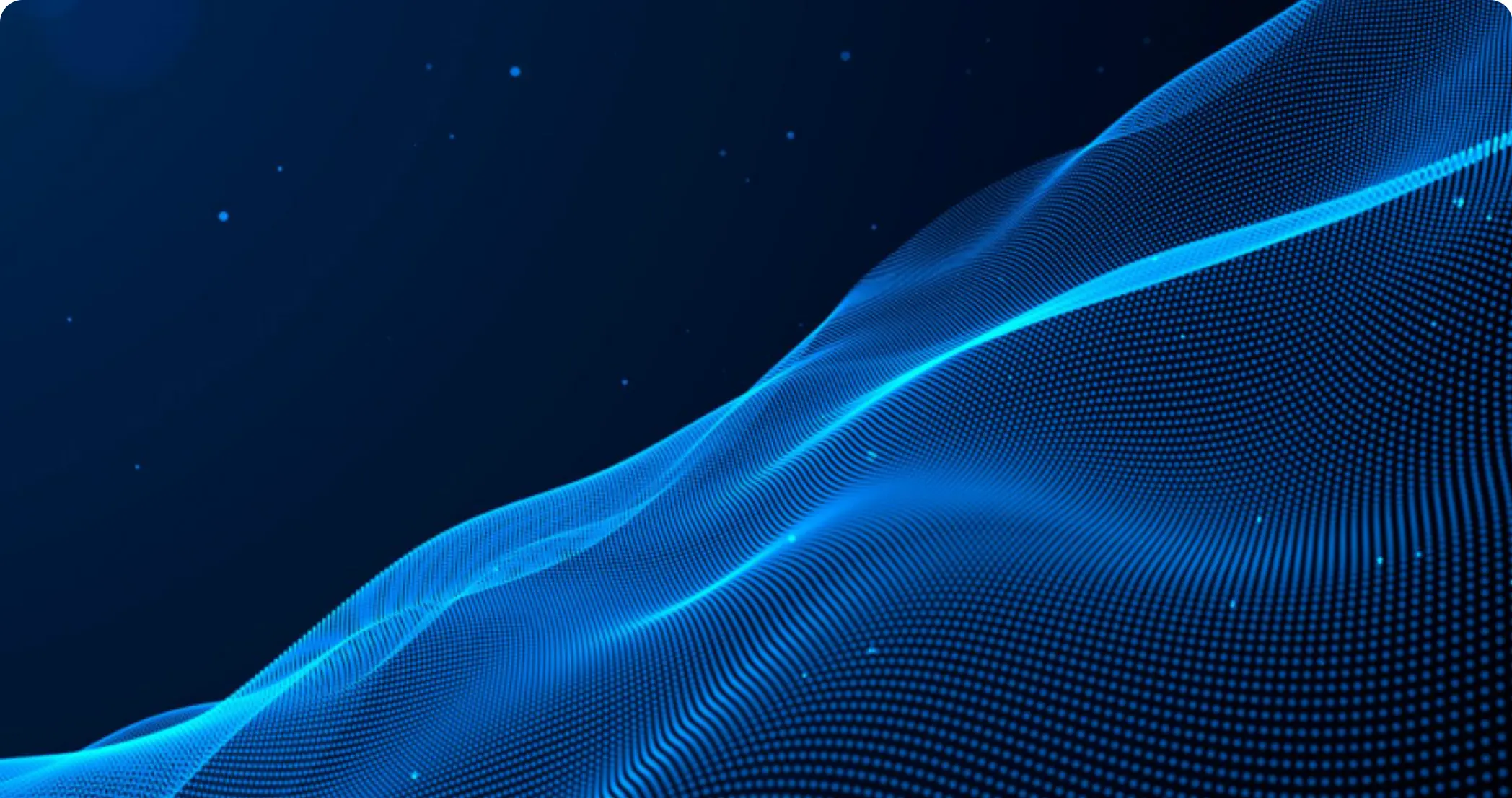
Enhanced Model Accuracy
Improve the accuracy and reliability of your ML models.

Scalable Operations
Scale your ML projects efficiently as your needs grow.
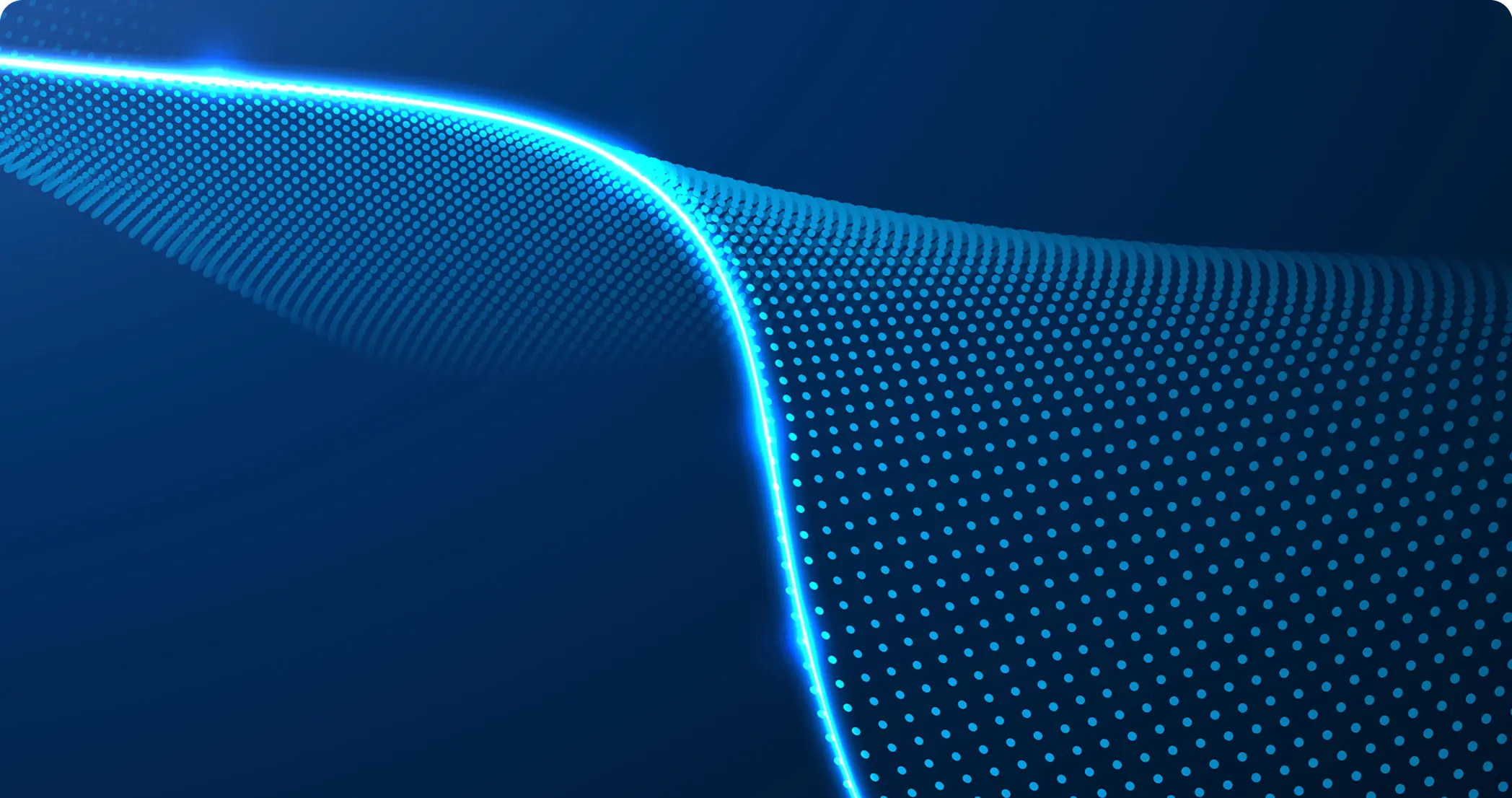
Expert Support
Gain from Opsio’s deep expertise in cloud and ML technologies.
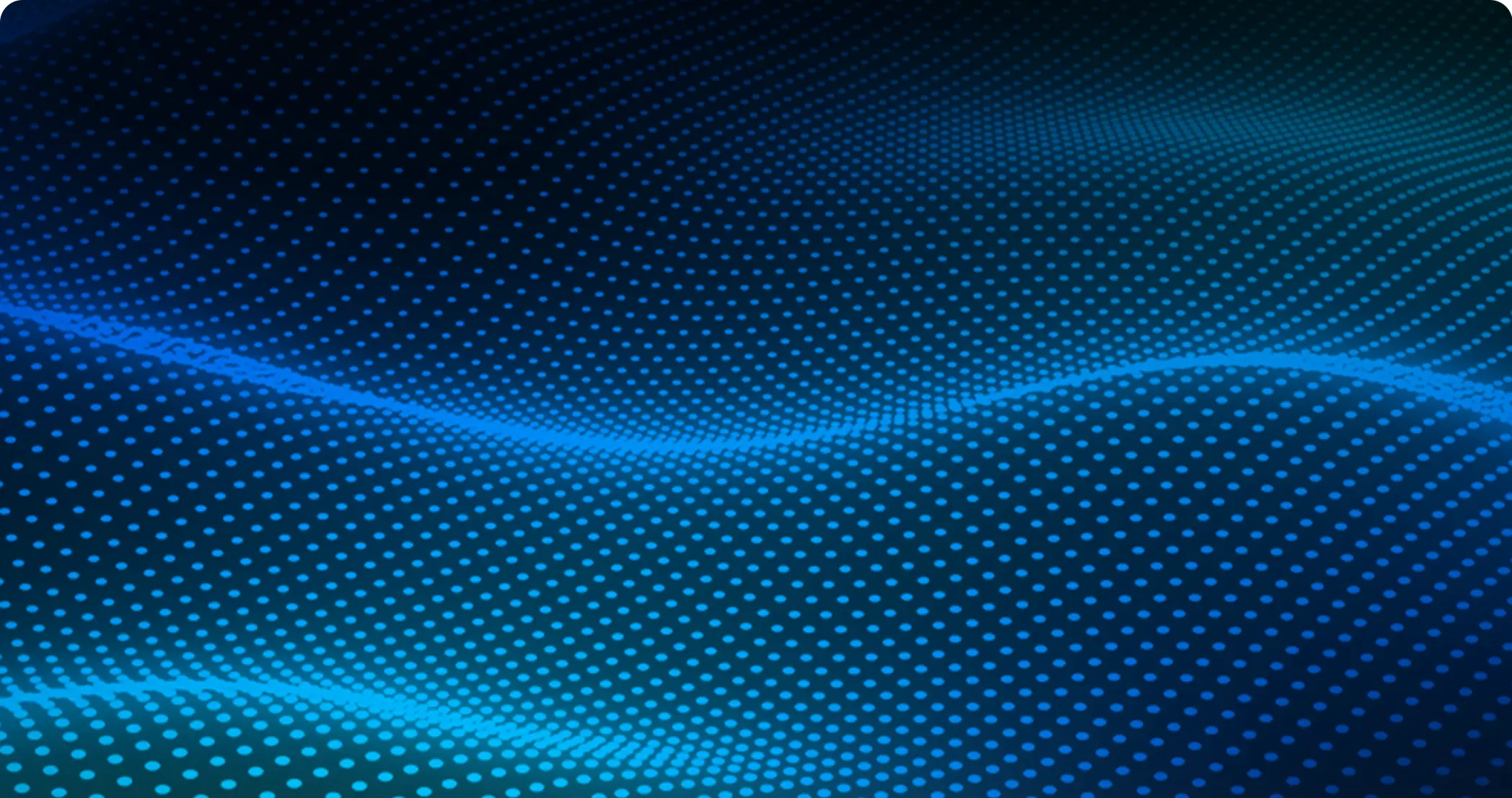
Innovative Edge
Stay ahead in the market with the latest MLOps advancements.
Machine Learning Operations (MLOps) Evolution: Your Opsio Roadmap To Success
Customer Introduction
Introductory meeting to explore needs, goals, and next steps.
Proposal
Onboarding
The shovel hits the ground through onboarding of our agreed service collaboration.

Assessment Phase
Compliance Activation
Run & Optimize
FAQ: Machine Learning Operations (MLOps)
What is MLOps platform?
“In the rapidly evolving landscape of artificial intelligence and machine learning, the term MLOps has emerged as a crucial concept. But what exactly is an MLOps platform? To understand this, we need to delve into the intricacies of machine learning operations (MLOps) and the role these platforms play in streamlining and enhancing the machine learning lifecycle.
MLOps, short for Machine Learning Operations, is a set of practices that aims to deploy and maintain machine learning models in production reliably and efficiently. It combines the principles of DevOps with machine learning, emphasizing collaboration, automation, and continuous improvement. An MLOps platform, therefore, is a comprehensive suite of tools and frameworks designed to facilitate these practices, ensuring that machine learning models can be developed, tested, deployed, and monitored effectively.
The importance of an MLOps platform cannot be overstated. In traditional software development, DevOps practices have revolutionized the way applications are built, tested, and deployed. Similarly, MLOps platforms are transforming the machine learning landscape by addressing the unique challenges associated with deploying machine learning models at scale.
One of the primary challenges in machine learning is the complexity of the model development lifecycle. This lifecycle typically involves data collection, data preprocessing, model training, model evaluation, and model deployment. Each of these stages can be fraught with difficulties, such as managing vast amounts of data, ensuring data quality, selecting appropriate algorithms, and fine-tuning model parameters. An MLOps platform provides a unified environment where data scientists and machine learning engineers can collaborate seamlessly, leveraging automation to streamline these processes.
Data management is a critical component of any MLOps platform. Machine learning models are only as good as the data they are trained on. An MLOps platform offers robust data management capabilities, allowing teams to ingest, store, and preprocess data efficiently. This ensures that models are trained on high-quality, relevant data, which is essential for achieving accurate predictions. Moreover, these platforms often include versioning features, enabling teams to track changes to datasets and models over time, facilitating reproducibility and auditability.
Model training and evaluation are other areas where an MLOps platform excels. Training machine learning models can be computationally intensive and time-consuming. An MLOps platform typically provides scalable computing resources, such as cloud-based GPU instances, to accelerate the training process. Additionally, these platforms offer tools for hyperparameter tuning, model selection, and performance evaluation, helping teams identify the best-performing models quickly and efficiently.
Once a model has been trained and evaluated, the next step is deployment. Deploying machine learning models to production environments can be a daunting task, especially when dealing with large-scale applications. An MLOps platform simplifies this process by providing deployment pipelines that automate the transition from development to production. These pipelines ensure that models are deployed consistently and reliably, reducing the risk of errors and downtime.
Monitoring and maintenance are also crucial aspects of the machine learning lifecycle. Models in production need to be continuously monitored to ensure they perform as expected. An MLOps platform offers monitoring tools that track key performance metrics, such as accuracy, latency, and throughput. If a model’s performance degrades over time, the platform can trigger alerts, enabling teams to take corrective action promptly. This proactive approach to monitoring helps maintain the reliability and effectiveness of machine learning models in production.
Collaboration is another significant advantage of using an MLOps platform. Machine learning projects often involve cross-functional teams, including data scientists, machine learning engineers, software developers, and business stakeholders. An MLOps platform fosters collaboration by providing a centralized workspace where team members can share code, data, and insights. This collaborative environment promotes transparency and alignment, ensuring that everyone is working towards the same goals.
Security is a paramount concern in any machine learning project. An MLOps platform incorporates security best practices to protect sensitive data and models. This includes features such as access controls, encryption, and audit logs. By safeguarding data and models, an MLOps platform helps organizations comply with regulatory requirements and mitigate the risk of data breaches.
In conclusion, an MLOps platform is an indispensable tool for organizations looking to harness the power of machine learning. By providing a comprehensive suite of tools and frameworks, these platforms streamline the machine learning lifecycle, from data management and model training to deployment and monitoring. They foster collaboration, enhance security, and ensure that machine learning models can be deployed and maintained efficiently and reliably. As the field of machine learning continues to evolve, the adoption of MLOps platforms will undoubtedly become increasingly prevalent, driving innovation and enabling organizations to unlock the full potential of their data.
In the rapidly evolving landscape of artificial intelligence and machine learning, the term MLOps has emerged as a crucial concept. But what exactly is an MLOps platform? To understand this, we need to delve into the intricacies of machine learning operations (MLOps) and the role these platforms play in streamlining and enhancing the machine learning lifecycle.
MLOps, short for Machine Learning Operations, is a set of practices that aims to deploy and maintain machine learning models in production reliably and efficiently. It combines the principles of DevOps with machine learning, emphasizing collaboration, automation, and continuous improvement. An MLOps platform, therefore, is a comprehensive suite of tools and frameworks designed to facilitate these practices, ensuring that machine learning models can be developed, tested, deployed, and monitored effectively.
The importance of an MLOps platform cannot be overstated. In traditional software development, DevOps practices have revolutionized the way applications are built, tested, and deployed. Similarly, MLOps platforms are transforming the machine learning landscape by addressing the unique challenges associated with deploying machine learning models at scale.
One of the primary challenges in machine learning is the complexity of the model development lifecycle. This lifecycle typically involves data collection, data preprocessing, model training, model evaluation, and model deployment. Each of these stages can be fraught with difficulties, such as managing vast amounts of data, ensuring data quality, selecting appropriate algorithms, and fine-tuning model parameters. An MLOps platform provides a unified environment where data scientists and machine learning engineers can collaborate seamlessly, leveraging automation to streamline these processes.
Data management is a critical component of any MLOps platform. Machine learning models are only as good as the data they are trained on. An MLOps platform offers robust data management capabilities, allowing teams to ingest, store, and preprocess data efficiently. This ensures that models are trained on high-quality, relevant data, which is essential for achieving accurate predictions. Moreover, these platforms often include versioning features, enabling teams to track changes to datasets and models over time, facilitating reproducibility and auditability.
Model training and evaluation are other areas where an MLOps platform excels. Training machine learning models can be computationally intensive and time-consuming. An MLOps platform typically provides scalable computing resources, such as cloud-based GPU instances, to accelerate the training process. Additionally, these platforms offer tools for hyperparameter tuning, model selection, and performance evaluation, helping teams identify the best-performing models quickly and efficiently.
Once a model has been trained and evaluated, the next step is deployment. Deploying machine learning models to production environments can be a daunting task, especially when dealing with large-scale applications. An MLOps platform simplifies this process by providing deployment pipelines that automate the transition from development to production. These pipelines ensure that models are deployed consistently and reliably, reducing the risk of errors and downtime.
Monitoring and maintenance are also crucial aspects of the machine learning lifecycle. Models in production need to be continuously monitored to ensure they perform as expected. An MLOps platform offers monitoring tools that track key performance metrics, such as accuracy, latency, and throughput. If a model’s performance degrades over time, the platform can trigger alerts, enabling teams to take corrective action promptly. This proactive approach to monitoring helps maintain the reliability and effectiveness of machine learning models in production.
Collaboration is another significant advantage of using an MLOps platform. Machine learning projects often involve cross-functional teams, including data scientists, machine learning engineers, software developers, and business stakeholders. An MLOps platform fosters collaboration by providing a centralized workspace where team members can share code, data, and insights. This collaborative environment promotes transparency and alignment, ensuring that everyone is working towards the same goals.
Security is a paramount concern in any machine learning project. An MLOps platform incorporates security best practices to protect sensitive data and models. This includes features such as access controls, encryption, and audit logs. By safeguarding data and models, an MLOps platform helps organizations comply with regulatory requirements and mitigate the risk of data breaches.
Scalability is another key aspect of MLOps platforms, particularly for organizations that deal with large volumes of data or require real-time predictions. These platforms are designed to handle the growing demands of machine learning workloads, providing the necessary infrastructure to scale operations seamlessly. Whether it’s scaling up to accommodate more data, more complex models, or more users, an MLOps platform ensures that the infrastructure can grow in tandem with the organization’s needs.
Furthermore, the integration capabilities of MLOps platforms are vital for creating a cohesive machine learning ecosystem. These platforms often support integration with various data sources, third-party tools, and cloud services, allowing organizations to build a customized and flexible machine learning pipeline. This interoperability ensures that teams can leverage the best tools for their specific needs, enhancing the overall efficiency and effectiveness of their machine learning initiatives.
In conclusion, an MLOps platform is an indispensable tool for organizations looking to harness the power of machine learning. By providing a comprehensive suite of tools and frameworks, these platforms streamline the machine learning lifecycle, from data management and model training to deployment and monitoring. They foster collaboration, enhance security, and ensure that machine learning models can be deployed and maintained efficiently and reliably. As the field of machine learning continues to evolve, the adoption of MLOps platforms will undoubtedly become increasingly prevalent, driving innovation and enabling organizations to unlock the full potential of their data. The future of machine learning lies in the seamless integration of these platforms into the core operations of businesses, paving the way for more intelligent, data-driven decision-making processes.”
What are MLOps?
In the rapidly evolving landscape of artificial intelligence (AI) and machine learning (ML), the term MLOps has emerged as a crucial concept. But what exactly are MLOps, and why are they so important for today’s data-driven enterprises? This blog post aims to delve deep into the world of MLOps, exploring its significance, components, and the transformative impact it has on the deployment and management of machine learning models.
The Genesis of MLOps
MLOps, short for Machine Learning Operations, is a set of practices that aim to deploy and maintain machine learning models in production reliably and efficiently. Drawing inspiration from DevOps, which focuses on software engineering, MLOps bridges the gap between data science and operations, facilitating seamless collaboration and integration. The primary goal is to streamline the ML lifecycle, from data preparation and model training to deployment and monitoring.
Why MLOps Matters
The advent of MLOps addresses several challenges that organizations face when scaling their machine learning efforts. Traditional ML workflows often encounter bottlenecks due to the lack of standardized processes, resulting in delayed deployments and increased operational costs. MLOps introduces automation, continuous integration, and continuous delivery (CI/CD) pipelines, enabling faster iterations and more robust models.
Moreover, MLOps enhances reproducibility and traceability. By maintaining detailed records of data versions, model parameters, and training processes, it becomes easier to reproduce results and debug issues. This is particularly critical in regulated industries where compliance and auditability are paramount.
Core Components of MLOps
To fully grasp the concept of MLOps, it’s essential to understand its core components, which collectively form a cohesive framework for managing the ML lifecycle.
Data Management
Effective data management is the cornerstone of any successful ML project. MLOps emphasizes the importance of data versioning, ensuring that datasets are consistently tracked and updated. This not only aids in reproducibility but also facilitates collaboration among data scientists. Tools like DVC (Data Version Control) and Delta Lake are commonly used to manage data pipelines and storage.
Model Training and Validation
Model training is an iterative process that involves selecting algorithms, tuning hyperparameters, and validating performance. MLOps introduces automation into this phase, leveraging tools like Kubernetes and Kubeflow to orchestrate training jobs on scalable infrastructure. Automated validation ensures that models meet predefined performance criteria before being deployed.
Continuous Integration and Continuous Deployment (CI/CD)
CI/CD pipelines are integral to MLOps, enabling seamless integration of new code and models into production. By automating the testing and deployment processes, CI/CD pipelines reduce the risk of errors and accelerate the release cycle. GitHub Actions, Jenkins, and GitLab CI are popular tools used to implement CI/CD in MLOps workflows.
Monitoring and Maintenance
Once deployed, ML models require continuous monitoring to ensure they perform as expected in real-world scenarios. MLOps incorporates monitoring tools like Prometheus and Grafana to track metrics such as accuracy, latency, and resource utilization. Additionally, drift detection mechanisms are employed to identify changes in data distribution that may impact model performance, prompting retraining or updates.
The Role of MLOps in Business Transformation
The integration of MLOps into business operations has far-reaching implications. For one, it democratizes access to machine learning, enabling teams with diverse skill sets to collaborate effectively. Data scientists, software engineers, and operations professionals can work together seamlessly, breaking down silos and fostering innovation.
Furthermore, MLOps enhances the scalability and reliability of ML systems. By automating repetitive tasks and standardizing workflows, organizations can deploy models at scale without compromising on quality. This is particularly beneficial for enterprises with large volumes of data and complex ML requirements.
Another significant advantage is the reduction in time-to-market. MLOps accelerates the development and deployment of ML models, allowing businesses to respond swiftly to market changes and customer needs. This agility is a competitive differentiator in industries such as finance, healthcare, and retail, where timely insights can drive strategic decision-making.
Challenges and Future Directions
Despite its numerous benefits, implementing MLOps is not without challenges. Organizations often grapple with the complexity of integrating diverse tools and technologies into a unified MLOps framework. Additionally, the cultural shift required to embrace MLOps can be daunting, necessitating buy-in from stakeholders across the organization.
Looking ahead, the future of MLOps is poised to be shaped by advancements in AI and cloud computing. Emerging trends such as serverless architectures, edge computing, and federated learning are likely to influence MLOps practices, driving further innovation. As the field continues to evolve, the focus will increasingly be on enhancing automation, improving model interpretability, and ensuring ethical AI deployment.
In summary, MLOps represents a paradigm shift in how machine learning models are developed, deployed, and maintained. By fostering collaboration, standardizing processes, and leveraging automation, MLOps empowers organizations to harness the full potential of AI, driving business transformation and delivering tangible value.
The Cultural Shift Towards MLOps Adoption
One of the key challenges in implementing MLOps is the cultural shift required within organizations. Embracing MLOps involves breaking down traditional silos between data science, engineering, and operations teams. It requires a shift towards a more collaborative and cross-functional approach, where stakeholders from different disciplines work together towards a common goal.
This cultural transformation can be daunting, as it often involves changing established processes and mindsets. It requires buy-in from leadership, clear communication across teams, and a willingness to embrace new ways of working. However, organizations that successfully navigate this cultural shift stand to benefit significantly from the adoption of MLOps.
By fostering a culture of collaboration and innovation, MLOps enables organizations to unlock the full potential of their machine learning initiatives. It encourages knowledge sharing, accelerates decision-making, and drives continuous improvement. Ultimately, the cultural shift towards MLOps adoption is essential for organizations looking to stay competitive in a data-driven world.”
What is MLOps and why do we need it?
“In the rapidly evolving landscape of technological innovation, Machine Learning Operations (MLOps) has emerged as a critical discipline, bridging the gap between machine learning (ML) models and their deployment in production environments. Understanding MLOps and its significance is essential for organizations aiming to harness the full potential of their data-driven initiatives. This blog post delves into the essence of MLOps, exploring its components, benefits, and the reasons behind its growing prominence.
MLOps, short for Machine Learning Operations, is a set of practices and tools designed to streamline the deployment, monitoring, and management of machine learning models in production. Drawing inspiration from DevOps, which revolutionized software development and operations, MLOps addresses the unique challenges posed by machine learning workflows. It integrates data engineering, model training, and model deployment into a cohesive framework, fostering collaboration among data scientists, ML engineers, and IT operations teams.
One of the primary reasons we need MLOps is the complexity inherent in machine learning projects. Unlike traditional software development, where code is deterministic and relatively static, machine learning models are dynamic and probabilistic. They rely heavily on data, which can change over time, leading to model drift and degraded performance. MLOps provides a structured approach to manage these challenges, ensuring models remain accurate and reliable over their lifecycle.
Data versioning is a crucial aspect of MLOps. In machine learning, the quality and consistency of data directly impact model performance. MLOps frameworks facilitate data versioning, enabling teams to track changes in datasets and reproduce experiments. This traceability is vital for debugging, auditing, and compliance purposes. By maintaining a clear lineage of data, organizations can ensure that models are trained and evaluated on the correct datasets, minimizing errors and enhancing reproducibility.
Another key component of MLOps is automated model training and deployment. Manual processes for training and deploying models are not only time-consuming but also prone to errors. MLOps leverages automation to streamline these tasks, reducing the time it takes to bring models from development to production. Continuous integration and continuous deployment (CI/CD) pipelines, adapted for machine learning, facilitate seamless transitions between different stages of the ML lifecycle. This automation enhances agility, allowing organizations to respond swiftly to changing business needs and market conditions.
Monitoring and observability are integral to MLOps. Once a model is deployed, it is crucial to monitor its performance in real-time. MLOps frameworks provide tools for tracking key metrics such as accuracy, latency, and resource utilization. By setting up alerts and dashboards, teams can quickly identify and address issues, ensuring models continue to deliver value. Additionally, observability extends to data pipelines, enabling proactive identification of data quality issues that could impact model performance.
Collaboration is another significant benefit of MLOps. Machine learning projects often involve diverse teams with different skill sets. Data scientists focus on model development, while ML engineers handle the technical aspects of deployment, and IT operations ensure the infrastructure is robust and scalable. MLOps fosters collaboration by providing a common platform and standardized processes, breaking down silos and enabling seamless communication. This collaborative approach accelerates the development cycle and improves the overall quality of machine learning solutions.
Scalability is a pressing concern for organizations deploying machine learning models. As the volume of data and the complexity of models increase, the infrastructure must scale accordingly. MLOps frameworks are designed to handle scalability challenges, providing tools for distributed training, model parallelism, and resource management. By leveraging cloud computing and containerization technologies, MLOps ensures that models can scale efficiently, accommodating growing demands without compromising performance.
Security and compliance are paramount in the deployment of machine learning models. MLOps incorporates best practices for securing data, models, and infrastructure. This includes encryption, access controls, and regular audits to ensure compliance with regulatory requirements. By embedding security into the MLOps pipeline, organizations can safeguard sensitive information and maintain trust with their stakeholders.
Furthermore, MLOps promotes experimentation and innovation. The iterative nature of machine learning requires constant experimentation to improve model performance. MLOps frameworks support this by providing tools for hyperparameter tuning, model versioning, and A/B testing. This experimental approach allows data scientists to explore different algorithms and techniques, driving continuous improvement and innovation.
In conclusion, MLOps is a transformative approach that addresses the unique challenges of deploying and managing machine learning models. By integrating data engineering, model training, and deployment into a cohesive framework, MLOps enhances collaboration, scalability, and security. It provides the automation, monitoring, and observability needed to ensure models remain accurate and reliable in production. As organizations continue to embrace machine learning, the adoption of MLOps will be crucial in unlocking the full potential of their data-driven initiatives.
As we delve deeper into the transformative power of MLOps, it’s essential to highlight the broader implications and future directions of this evolving discipline. Beyond the immediate benefits to machine learning workflows, MLOps is poised to redefine how organizations approach data science and artificial intelligence (AI) at a strategic level.
The Role of MLOps in Democratizing AI
One of the most profound impacts of MLOps is its potential to democratize AI. Traditionally, the deployment and management of machine learning models required specialized knowledge and significant resources, limiting access to large enterprises with dedicated data science teams. However, MLOps frameworks simplify and standardize these processes, making them accessible to a broader range of organizations, including small and medium-sized enterprises (SMEs).
By lowering the barriers to entry, MLOps enables more organizations to leverage AI for decision-making, innovation, and competitive advantage. This democratization fosters a more inclusive AI ecosystem, where diverse industries and sectors can benefit from advanced analytics and machine learning capabilities.
Enhancing Ethical AI Practices
Ethical considerations are becoming increasingly important in the field of AI. Issues such as bias, fairness, transparency, and accountability are at the forefront of discussions about the responsible use of machine learning. MLOps can play a crucial role in enhancing ethical AI practices by embedding ethical guidelines and checks into the ML lifecycle.
For instance, MLOps frameworks can incorporate bias detection tools that analyze model outputs for potential biases and ensure fairness across different demographic groups. Transparency can be achieved through comprehensive documentation and explainability features, allowing stakeholders to understand how models make decisions. Accountability is reinforced by maintaining detailed logs and audit trails, which are essential for compliance and governance.
The Intersection of MLOps and Edge Computing
As the Internet of Things (IoT) continues to grow, the intersection of MLOps and edge computing is an exciting area of development. Edge computing involves processing data closer to the source, such as sensors or local devices, rather than relying solely on centralized cloud infrastructure. This approach reduces latency and enables real-time decision-making, which is critical for applications like autonomous vehicles, smart cities, and industrial automation.
MLOps frameworks are evolving to support edge deployment, allowing models to be trained in the cloud and then deployed and managed on edge devices. This seamless integration ensures that machine learning models can operate efficiently in environments with limited connectivity and resources, expanding the reach and applicability of AI solutions.
Future Trends and Innovations in MLOps
The future of MLOps is bright, with several emerging trends and innovations set to shape the landscape:
1. AI-Driven MLOps: The application of AI to optimize MLOps processes themselves, such as automated hyperparameter tuning, anomaly detection in model performance, and intelligent resource allocation, will further enhance efficiency and effectiveness.
2. Federated Learning: This approach involves training models across decentralized devices or servers while keeping data localized. MLOps frameworks will need to adapt to support federated learning, ensuring privacy and security while enabling collaborative model development.
3. Explainable AI (XAI): As the demand for transparency and interpretability grows, MLOps will increasingly incorporate XAI techniques, providing insights into model behavior and decision-making processes.
4. Green AI: Sustainability is becoming a critical consideration. MLOps can contribute to green AI initiatives by optimizing resource usage, reducing energy consumption, and promoting environmentally friendly practices in model training and deployment.
Conclusion
In summary, MLOps is not just a set of tools and practices; it is a paradigm shift that is reshaping the landscape of machine learning and AI. By addressing the complexities of deploying and managing machine learning models, MLOps empowers organizations to harness the full potential of their data-driven initiatives. Its role in democratizing AI, enhancing ethical practices, and supporting cutting-edge technologies like edge computing underscores its significance in the broader AI ecosystem.
As we look to the future, the continued evolution of MLOps will drive innovation, efficiency, and inclusivity in AI, ensuring that the benefits of machine learning are accessible to all. Organizations that embrace MLOps will be well-positioned to lead in the era of intelligent automation, making data-driven decisions that propel them toward sustained success and growth.”
What does MLOps do?
In the rapidly evolving world of artificial intelligence (AI) and machine learning (ML), the term MLOps has garnered significant attention. But what exactly does MLOps do? To grasp its significance, it’s essential to dive into the intricacies of MLOps, its functions, and its impact on the machine learning lifecycle.
The Genesis of MLOps
MLOps, a portmanteau of Machine Learning and Operations, is an emerging discipline that aims to streamline and automate the end-to-end machine learning lifecycle. Drawing parallels with DevOps—an established methodology in software development that emphasizes collaboration between development and operations—MLOps applies similar principles to the world of machine learning.
Enhancing Collaboration and Efficiency
One of the primary roles of MLOps is to foster collaboration between data scientists, ML engineers, and operations teams. Traditionally, these teams have operated in silos, leading to inefficiencies, miscommunications, and prolonged project timelines. MLOps bridges these gaps by creating a unified framework that encourages seamless communication and collaboration.
By integrating various tools and practices, MLOps ensures that models transition smoothly from development to production. This integration is vital because it reduces the time taken to deploy models, thereby accelerating the delivery of AI-driven solutions.
Automating the Machine Learning Lifecycle
Automation is at the heart of MLOps. The machine learning lifecycle comprises several stages, including data collection, preprocessing, model training, validation, deployment, and monitoring. Each of these stages involves complex tasks that can be time-consuming and prone to human error.
MLOps leverages automation to streamline these tasks. For instance, automated data pipelines can handle data ingestion, cleaning, and transformation, ensuring that data scientists have access to high-quality data. Automated model training and hyperparameter tuning can significantly reduce the time and effort required to develop robust models. Once models are trained, automated deployment pipelines ensure that they are seamlessly integrated into production environments.
Ensuring Model Reliability and Scalability
In production environments, the reliability and scalability of machine learning models are paramount. MLOps addresses these concerns by implementing best practices for model monitoring, versioning, and rollback mechanisms.
Model monitoring involves continuously tracking the performance of deployed models to detect anomalies, data drift, or degradation in accuracy. This proactive approach allows teams to take corrective actions promptly, ensuring that models remain reliable and performant.
Versioning is another critical aspect of MLOps. By maintaining a version history of models, data, and code, teams can easily trace the lineage of any model and reproduce results. This traceability is crucial for debugging, auditing, and compliance purposes.
Scalability is achieved through the use of containerization and orchestration technologies like Docker and Kubernetes. These technologies enable models to be deployed in scalable, distributed environments, ensuring that they can handle varying workloads and user demands.
Facilitating Continuous Integration and Continuous Deployment (CI/CD)
Continuous Integration and Continuous Deployment (CI/CD) are fundamental principles of MLOps. CI/CD pipelines automate the process of integrating code changes, testing, and deploying models, ensuring that updates are delivered rapidly and reliably.
In the context of MLOps, CI/CD pipelines encompass not only code but also data and models. This holistic approach ensures that any changes to data preprocessing scripts, model architectures, or hyperparameters are automatically tested and validated before being deployed.
By adopting CI/CD practices, organizations can achieve faster iteration cycles, reduce the risk of introducing errors, and maintain a high level of quality in their machine learning solutions.
Promoting Reproducibility and Governance
Reproducibility and governance are critical concerns in the field of machine learning. Reproducibility refers to the ability to consistently reproduce the results of a model using the same data and code. Governance involves establishing policies and procedures to ensure that machine learning practices comply with regulatory and ethical standards.
MLOps addresses these concerns by implementing robust version control, documentation, and auditing practices. By tracking changes to data, code, and models, MLOps ensures that experiments are reproducible and that results can be independently verified.
Governance is further enhanced through the use of model explainability tools, which provide insights into how models make decisions. This transparency is essential for building trust with stakeholders and ensuring compliance with regulations such as GDPR and CCPA.
Driving Business Value
Ultimately, the goal of MLOps is to drive business value by enabling organizations to harness the full potential of machine learning. By streamlining the machine learning lifecycle, enhancing collaboration, and ensuring reliability and scalability, MLOps empowers organizations to deliver AI-driven solutions more efficiently and effectively.
This increased efficiency translates into tangible benefits, such as reduced time-to-market, improved customer experiences, and the ability to make data-driven decisions with greater confidence. As organizations continue to invest in AI and ML, the adoption of MLOps practices will be instrumental in realizing the full potential of these technologies.
In conclusion, MLOps is a transformative discipline that addresses the unique challenges of the machine learning lifecycle. By fostering collaboration, automating processes, ensuring reliability and scalability, facilitating CI/CD, and promoting reproducibility and governance, MLOps empowers organizations to deliver high-quality AI-driven solutions that drive business value. As the field continues to evolve, the importance of MLOps will only grow, making it an essential component of any successful machine learning strategy.
The Future of MLOps: Emerging Trends and Innovations
As MLOps continues to evolve, several emerging trends and innovations are shaping its future. These advancements promise to further enhance the capabilities of MLOps, making it an even more integral part of the machine learning landscape.
Integration with Edge Computing
One of the most exciting developments in the MLOps space is the integration with edge computing. Edge computing involves processing data closer to where it is generated, such as on IoT devices or local servers, rather than relying solely on centralized cloud infrastructure. This approach reduces latency and bandwidth usage, enabling real-time decision-making and analytics.
MLOps can facilitate the deployment and management of machine learning models at the edge. By leveraging edge computing, organizations can deploy models in environments where immediate data processing is crucial, such as in autonomous vehicles, smart cities, and industrial IoT applications. This integration ensures that models remain responsive and effective even in distributed and resource-constrained environments.
Enhanced Model Explainability and Interpretability
As machine learning models become more complex, understanding how they make decisions becomes increasingly challenging. Enhanced model explainability and interpretability are critical for building trust with stakeholders and ensuring compliance with regulatory requirements.
MLOps is evolving to include advanced explainability tools that provide insights into model behavior. Techniques such as SHAP (SHapley Additive exPlanations) and LIME (Local Interpretable Model-agnostic Explanations) are being integrated into MLOps pipelines to offer detailed explanations of model predictions. This transparency not only aids in debugging and auditing but also helps in addressing ethical concerns related to AI decision-making.
Federated Learning and Privacy-Preserving Techniques
Data privacy and security are paramount concerns in the machine learning domain. Federated learning is an emerging technique that addresses these concerns by enabling model training across decentralized data sources without requiring data to be centralized. This approach preserves data privacy while still allowing for collaborative model development.
MLOps platforms are beginning to incorporate federated learning capabilities, allowing organizations to train models on sensitive data without compromising privacy. Additionally, privacy-preserving techniques such as differential privacy and homomorphic encryption are being integrated into MLOps workflows to ensure that data remains secure throughout the machine learning lifecycle.
AI-Driven MLOps
The concept of using AI to manage AI is gaining traction, leading to the development of AI-driven MLOps. This approach leverages machine learning algorithms to optimize various aspects of the MLOps pipeline, such as automated hyperparameter tuning, anomaly detection in model performance, and predictive maintenance of infrastructure.
By incorporating AI-driven automation, MLOps platforms can become more adaptive and responsive to changing conditions. This self-optimizing capability reduces the need for manual intervention, allowing data scientists and engineers to focus on higher-level tasks and innovation.
Democratization of MLOps
As the demand for machine learning solutions grows, there is a push towards democratizing MLOps to make it accessible to a broader audience. Low-code and no-code platforms are emerging, enabling individuals with limited technical expertise to build, deploy, and manage machine learning models.
These platforms provide intuitive interfaces and pre-built components that simplify the MLOps process. By lowering the barrier to entry, democratized MLOps empowers a diverse range of professionals to leverage machine learning in their respective fields, fostering innovation and accelerating AI adoption across industries.
Integration with DevSecOps
Security is a critical aspect of any software development lifecycle, and the integration of security practices into DevOps has given rise to DevSecOps. Similarly, MLOps is beginning to incorporate security measures throughout the machine learning lifecycle, leading to the concept of MLOpsSec.
MLOpsSec involves embedding security practices into every stage of the MLOps pipeline, from data collection and preprocessing to model deployment and monitoring. This proactive approach ensures that machine learning models are robust against adversarial attacks, data breaches, and other security threats, safeguarding both the models and the data they rely on.
Conclusion
The future of MLOps is bright, with numerous innovations and trends poised to enhance its capabilities and impact. By integrating with edge computing, enhancing model explainability, adopting privacy-preserving techniques, leveraging AI-driven automation, democratizing access, and incorporating security practices, MLOps is set to become an even more powerful tool for organizations seeking to harness the potential of machine learning.
As these trends continue to evolve, MLOps will play a crucial role in driving the next wave of AI advancements, enabling organizations to deliver smarter, more efficient, and more secure AI-driven solutions. Embracing these innovations will be key to staying ahead in the competitive landscape of artificial intelligence and machine learning.”
What is MLOps pipeline?
“In the ever-evolving field of machine learning, the term MLOps has emerged as a crucial concept for organizations aiming to operationalize their machine learning models efficiently. But what exactly is an MLOps pipeline, and why is it important for your business? In this blog post, we’ll dive deep into the intricacies of MLOps pipelines, their components, and their significance in modern data-driven enterprises.
MLOps, short for Machine Learning Operations, is a set of practices that aim to deploy and maintain machine learning models in production reliably and efficiently. It is an intersection of machine learning, DevOps, and data engineering, aiming to streamline the deployment, monitoring, and management of ML models. The MLOps pipeline is a structured approach to automate and manage the lifecycle of machine learning models, from development to deployment and beyond.
The journey of a machine learning model from conception to production is fraught with challenges. Data scientists and machine learning engineers often face issues related to data quality, reproducibility, scalability, and monitoring. An MLOps pipeline addresses these challenges by providing a systematic framework that ensures the smooth transition of models from development to production.
One of the primary components of an MLOps pipeline is data ingestion and preprocessing. Raw data is rarely in a format suitable for machine learning. It often requires cleaning, transformation, and augmentation to make it usable. An MLOps pipeline automates these tasks, ensuring that data is consistently prepared for training and evaluation. This step not only saves time but also ensures that the data used for training is of high quality, leading to more accurate models.
Once the data is prepared, the next step in the MLOps pipeline is model training. This involves selecting the right algorithms, tuning hyperparameters, and training the model on the prepared data. An MLOps pipeline provides a controlled environment for these tasks, ensuring that experiments are reproducible and results are consistent. This is particularly important in collaborative settings where multiple data scientists may be working on the same project.
After a model is trained, it needs to be validated and tested. This involves evaluating the model’s performance on a separate validation dataset to ensure that it generalizes well to new, unseen data. An MLOps pipeline automates this process, providing metrics and visualizations that help data scientists assess the model’s performance. This step is crucial for identifying potential issues such as overfitting or underfitting, which can significantly impact the model’s effectiveness in production.
Once a model passes validation, it is ready for deployment. Deploying machine learning models is a complex task that involves integrating the model with existing systems, scaling it to handle large volumes of data, and ensuring that it meets performance requirements. An MLOps pipeline simplifies this process by providing tools and frameworks that automate deployment tasks. This ensures that models are deployed quickly and reliably, reducing the time-to-value for machine learning projects.
Monitoring and maintenance are also critical components of an MLOps pipeline. Once a model is deployed, it needs to be continuously monitored to ensure that it performs as expected. This involves tracking metrics such as accuracy, latency, and resource usage, and identifying any anomalies that may indicate issues with the model or the data. An MLOps pipeline provides tools for automated monitoring and alerting, ensuring that potential issues are detected and addressed promptly.
Another important aspect of an MLOps pipeline is versioning and reproducibility. In a fast-paced development environment, it is crucial to keep track of different versions of data, code, and models. An MLOps pipeline provides version control mechanisms that ensure that every change is documented and can be traced back to its origin. This makes it easier to reproduce experiments, debug issues, and collaborate with team members.
Scalability is another key benefit of an MLOps pipeline. As machine learning models become more complex and data volumes increase, it becomes challenging to manage resources and ensure that models perform efficiently. An MLOps pipeline provides tools for scaling models horizontally and vertically, ensuring that they can handle large volumes of data and requests without compromising performance.
Security and compliance are also important considerations in an MLOps pipeline. Machine learning models often handle sensitive data, and it is crucial to ensure that this data is protected. An MLOps pipeline provides mechanisms for securing data, managing access controls, and ensuring compliance with regulatory requirements. This helps organizations build trust with their customers and stakeholders, ensuring that their data is handled responsibly.
In summary, an MLOps pipeline is a comprehensive framework that automates and manages the lifecycle of machine learning models. It addresses the challenges of data preparation, model training, validation, deployment, monitoring, versioning, scalability, and security. By providing a structured approach to these tasks, an MLOps pipeline ensures that machine learning models are developed, deployed, and maintained efficiently, enabling organizations to unlock the full potential of their data and drive business value.
In the ever-evolving field of machine learning, the term MLOps has emerged as a crucial concept for organizations aiming to operationalize their machine learning models efficiently. But what exactly is an MLOps pipeline, and why is it important for your business? In this blog post, we’ll dive deep into the intricacies of MLOps pipelines, their components, and their significance in modern data-driven enterprises.
MLOps, short for Machine Learning Operations, is a set of practices that aim to deploy and maintain machine learning models in production reliably and efficiently. It is an intersection of machine learning, DevOps, and data engineering, aiming to streamline the deployment, monitoring, and management of ML models. The MLOps pipeline is a structured approach to automate and manage the lifecycle of machine learning models, from development to deployment and beyond.
The journey of a machine learning model from conception to production is fraught with challenges. Data scientists and machine learning engineers often face issues related to data quality, reproducibility, scalability, and monitoring. An MLOps pipeline addresses these challenges by providing a systematic framework that ensures the smooth transition of models from development to production.
One of the primary components of an MLOps pipeline is data ingestion and preprocessing. Raw data is rarely in a format suitable for machine learning. It often requires cleaning, transformation, and augmentation to make it usable. An MLOps pipeline automates these tasks, ensuring that data is consistently prepared for training and evaluation. This step not only saves time but also ensures that the data used for training is of high quality, leading to more accurate models.
Once the data is prepared, the next step in the MLOps pipeline is model training. This involves selecting the right algorithms, tuning hyperparameters, and training the model on the prepared data. An MLOps pipeline provides a controlled environment for these tasks, ensuring that experiments are reproducible and results are consistent. This is particularly important in collaborative settings where multiple data scientists may be working on the same project.
After a model is trained, it needs to be validated and tested. This involves evaluating the model’s performance on a separate validation dataset to ensure that it generalizes well to new, unseen data. An MLOps pipeline automates this process, providing metrics and visualizations that help data scientists assess the model’s performance. This step is crucial for identifying potential issues such as overfitting or underfitting, which can significantly impact the model’s effectiveness in production.
Once a model passes validation, it is ready for deployment. Deploying machine learning models is a complex task that involves integrating the model with existing systems, scaling it to handle large volumes of data, and ensuring that it meets performance requirements. An MLOps pipeline simplifies this process by providing tools and frameworks that automate deployment tasks. This ensures that models are deployed quickly and reliably, reducing the time-to-value for machine learning projects.
Monitoring and maintenance are also critical components of an MLOps pipeline. Once a model is deployed, it needs to be continuously monitored to ensure that it performs as expected. This involves tracking metrics such as accuracy, latency, and resource usage, and identifying any anomalies that may indicate issues with the model or the data. An MLOps pipeline provides tools for automated monitoring and alerting, ensuring that potential issues are detected and addressed promptly.
Another important aspect of an MLOps pipeline is versioning and reproducibility. In a fast-paced development environment, it is crucial to keep track of different versions of data, code, and models. An MLOps pipeline provides version control mechanisms that ensure that every change is documented and can be traced back to its origin. This makes it easier to reproduce experiments, debug issues, and collaborate with team members.
Scalability is another key benefit of an MLOps pipeline. As machine learning models become more complex and data volumes increase, it becomes challenging to manage resources and ensure that models perform efficiently. An MLOps pipeline provides tools for scaling models horizontally and vertically, ensuring that they can handle large volumes of data and requests without compromising performance.
Security and compliance are also important considerations in an MLOps pipeline. Machine learning models often handle sensitive data, and it is crucial to ensure that this data is protected. An MLOps pipeline provides mechanisms for securing data, managing access controls, and ensuring compliance with regulatory requirements. This helps organizations build trust with their customers and stakeholders, ensuring that their data is handled responsibly.
In summary, an MLOps pipeline is a comprehensive framework that automates and manages the lifecycle of machine learning models. It addresses the challenges of data preparation, model training, validation, deployment, monitoring, versioning, scalability, and security. By providing a structured approach to these tasks, an MLOps pipeline ensures that machine learning models are developed, deployed, and maintained efficiently, enabling organizations to unlock the full potential of their data and drive business value.
The Role of Collaboration and Communication in MLOps
Effective collaboration and communication are vital in the MLOps framework. Machine learning projects often require the combined expertise of data scientists, data engineers, DevOps professionals, and business stakeholders. An MLOps pipeline fosters collaboration by providing a unified platform where team members can share insights, track progress, and align their efforts towards common goals. Tools such as version control systems, automated documentation, and shared dashboards ensure that every team member has access to the latest information and can contribute effectively to the project.
Continuous Integration and Continuous Deployment (CI/CD) in MLOps
Continuous Integration and Continuous Deployment (CI/CD) are core principles borrowed from DevOps that are equally applicable in MLOps. CI/CD pipelines automate the process of integrating code changes, testing, and deploying models, ensuring that updates are delivered quickly and reliably. This automation reduces the risk of human error, speeds up the development cycle, and allows for rapid experimentation and iteration. By incorporating CI/CD practices, organizations can ensure that their machine learning models are always up-to-date and can respond swiftly to changing business needs.
The Future of MLOps: Trends and Innovations
The field of MLOps is rapidly evolving, with new tools and technologies emerging to address the growing complexity of machine learning projects. One significant trend is the integration of artificial intelligence (AI) and machine learning (ML) with traditional DevOps practices, creating a more holistic approach known as AIOps. AIOps leverages AI to enhance the automation, monitoring, and management of IT operations, providing deeper insights and more proactive issue resolution.
Another emerging trend is the use of Kubernetes and containerization in MLOps. Kubernetes provides a scalable and flexible platform for deploying machine learning models, allowing organizations to manage their ML workloads more efficiently. Containerization ensures that models run consistently across different environments, reducing the risk of deployment issues.
Furthermore, the rise of explainable AI (XAI) is driving the need for MLOps pipelines to include mechanisms for interpreting and explaining model decisions. This is particularly important in regulated industries where transparency and accountability are crucial. By incorporating XAI tools, organizations can build trust in their models and ensure that they comply with regulatory requirements.
Conclusion
In conclusion, MLOps is not just a set of tools and practices; it is a paradigm shift that transforms how organizations develop, deploy, and manage machine learning models. By embracing MLOps, businesses can overcome the challenges of operationalizing ML models, ensuring that they deliver consistent, reliable, and scalable results. As the field continues to evolve, staying abreast of the latest trends and innovations will be key to maintaining a competitive edge in the data-driven economy. Whether you are just starting your MLOps journey or looking to enhance your existing practices, investing in a robust MLOps pipeline will be instrumental in unlocking the full potential of your machine learning initiatives.”